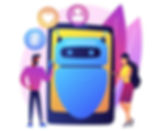
It’s tempting to assume that a solution built on generative AI can work like a plug-and-play system.
All you have to do is feed it data from your customer support software, like your previous customer interactions or help articles. It’ll magically train itself and learn from that knowledge, and it’ll update itself based on any new information from tickets.
Isn’t that the definition of machine learning?
Unsurprisingly, it doesn’t work that way in practice. AI chatbots require extensive training and considerable maintenance effort to achieve good results.
And feedback is the cornerstone of how you do that.
Setting your AI chatbot up for success
Almost 50% of customer support teams are already using AI. However, there are some basics you need to know when implementing AI for customer service:
Define clear objectives and use cases. You should have a clear idea of where it fits in your overall customer support strategy: which cases you want to use it for and why, the impact you want it to have on your customers, and how it will change your CS team.
Establish baseline performance metrics. What do your customers expect? How complex is your product? AI is great at handling repetitive, relatively straightforward customer requests. It’s not as good at handling complicated issues that require serious troubleshooting. AI can still have a role to play in complex tech support, but the metrics you’ll use to gauge its success might be different.
Ensure a comprehensive and accurate knowledge base. The number one piece of advice that can reduce AI hallucinations is to invest in maintaining your knowledge base. AI chatbots are much easier to train if you have a great base of content to work from,
Implement proper escalation protocols. Designing an elegant and effortless handoff from the AI to your human team significantly improves the overall customer experience — and ensures your customers never waste time interacting with your bot.
Collect and action feedback. AI solutions typically need more context than your human agents have. . They might also struggle to make connections that might be implicitly obvious to you. Everything needs to be documented and fed as training data.
Mechanisms to collect feedback
In most cases, you’ll want to start with a small selection of questions you want to automate. Over time, you’ll see where misunderstandings happen only through real customer interactions.
Customer feedback helps you quickly uncover those knowledge gaps. It also shows you what customers actually want and need, so you can tailor the chatbot’s responses accordingly.
The first challenge is how to collect and analyze feedback from those interactions. You can:
Do rigorous QA before launching it. Have a dedicated group of people rigorously test and challenge the AI chatbot's responses and capabilities, identifying potential weaknesses and areas for improvement.
Collect feedback via customer surveys. Implement post-interaction surveys measuring Customer Satisfaction (CSAT) and Customer Effort Score (CES) to gain direct insights into user experiences.
Analyze interactions manually via your team. Have your CS agents regularly review chatbot conversations to identify nuanced issues, misunderstandings, or opportunities for improvement that automated systems might miss. Most teams start with this. At the next stage, the team usually monitors only some cases based on a set of criteria (e.g., when customers provide a negative rating or the bot sends multiple replies).
Use (automated) feedback analysis tools. Leverage AI-powered analytics tools to process large volumes of chatbot interactions, automatically categorizing issues, detecting patterns, and highlighting areas that require attention. Note that these will often require their own training effort, so they’re often only worth it if you’re dealing with large volumes of data.
How to create a robust feedback loop for your chatbot
The overarching steps to creating a chatbot feedback loop are simple. You need to:
Collect feedback from different sources.
Translate that feedback into action by updating help articles or providing additional training to the chatbot.
Testing or checking that the AI chatbot can now handle that case better.
It becomes more complicated when you’re dealing with larger volumes of data or across multiple team members. Then, you have to prioritize the feedback and ensure the team is aligned and consistent.
These are the four most impactful areas to focus on when working with feedback.
Gathering insights from the support team
Whether you’re using a model like Knowledge-Centered Service or not, frontline support agents are the best source of feedback for any AI implementation.
The great thing about systems like KCS is that they encourage everyone in the team to regularly share, capture, and flag knowledge – making the transition to doing that with AI chatbot answers a lot more natural.
Either way, it’s essential to implement a system that lets them flag chatbot answers. It might take some weeks until that happens naturally as part of their regular workflow when interacting with customers. Leveraging their collective experience will help you improve the chatbot faster.
You can also use a system where they immediately categorize the feedback (e.g. incorrect information, misunderstanding the customer query, etc.) to make prioritizing those points of feedback easier.
Other methods to work on this could be:
Encouraging agents to suggest new responses as part of their workflow.
Analyzing patterns in conversations that get routed or escalated to your team.
This makes it easy for agents to adapt the chatbot’s responses directly when they notice issues rather than just flag them. Note that this requires a higher level of training across the team, so you might need a dedicated channel or meeting to ensure regular communication.
Actioning feedback on help articles
Great knowledge management processes lead to high-performing chatbots. That’s because out-of-date knowledge is noticed and corrected quickly.
Duplicated feedback or having agents flag the same issue multiple times is a lot of wasted effort.
That’s where using tools like the Help Center Manager (for Zendesk users) can have a massive impact. It allows you to collect customer feedback on articles – which you can make sure gets applied quickly so that the chatbot doesn’t repeat the same mistakes. It can also make it easier to apply bulk updates (with features like finding and replacing UI terms, correcting broken links, or applying automatic translations).
It’s often a good idea to:
Prioritize articles based on feedback volume and impact. A good way to reduce the risk of having out-of-date articles impact a lot of people is to update the ones that are most commonly referred to in the chatbot.
Implement a version control system for help articles. This is especially helpful if you collaborate across the team to train the AI chatbot, so a team member can check and confirm that an update was applied without necessarily having to ask.
Monitor the impact of changes on customer satisfaction. How easy this is depends on your tools. Some, like Help Center Manager, let you track the helpfulness score after an update so you can see if your changes have an impact.
Audit and update the entire knowledge base regularly. Regular help center audits are incredibly useful for culling content that is no longer helpful. Feeding the chatbot as many different data sources as possible is tempting, but some content leads to surprising outcomes. For example, if you maintain a change log for your customers or have an article listing feature requests, bots often assume that you provide certain features (when you don’t). Not all data is good data for a chatbot.
Using reports to triage and prioritize improvements
You can start by manually reviewing every single interaction with the AI chatbot. Once you hit a certain accuracy rate (say around 90+%), it can start feeling like reviewing every interaction is not the best use of your team’s time.
At that point, creating a few reports will help you identify the remaining 10% of inaccurate responses and deal with that feedback quickly.
Set up dashboards that surface negative ratings or high replies. These are often good indicators of where the chatbot can improve.
Implement sentiment analysis on customer feedback. Sentiment analysis is especially useful in highlighting urgent or escalated cases and proactively implementing a different process for these.
Create weighted scoring systems for prioritizing issues. Say you’re collecting customer feedback in a spreadsheet. You could have an automated set of criteria that helps you identify the most urgent issues to correct.
Establish thresholds for automatic escalation of critical issues. In some cases, an AI chatbot will be most helpful in collecting information and passing the case off to your team. The best way to handle these is to make the escalation process efficient and painless.
Level up your knowledge management
Having a high-performing AI chatbot requires a proactive approach to feedback and knowledge management.
At the heart of AI-driven support systems is a well-maintained knowledge base.
Ensuring that your help articles are current, accurate, and easily accessible allows your chatbot to perform at its best.
At Swifteq, we care about developing these tools. We’ve created a suite of Zendesk apps for customer support teams of all sizes to improve their knowledge management or automate parts of their workflows.
If you want to level up your team today, sign up for a 14-day free trial.